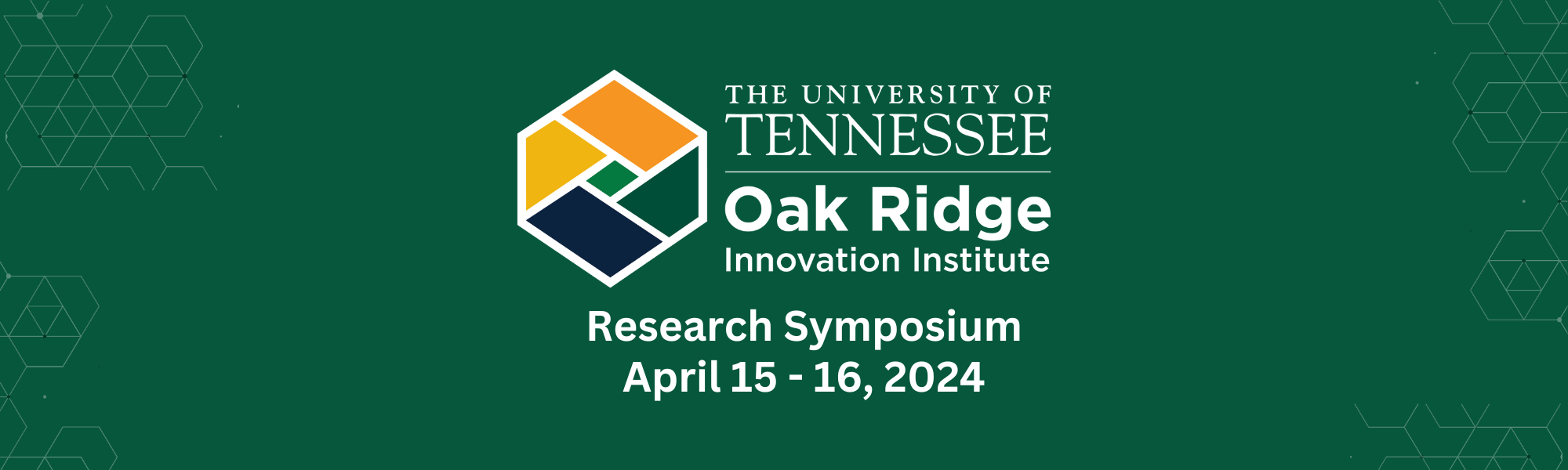
Faculty Presentations
April 15 & 16
UT Student Union
UT-ORII is expanding UT-Oak Ridge National Laboratory (ORNL) collaborative research through grant funding for teams that include both university faculty and ORNL scientists. Faculty research presentations focus on topics within the institute’s current convergent research initiatives; clean manufacturing and advanced materials, and energy storage and transportation. Presentations are held both April 15 and 16 with morning and afternoon sessions.
Monday April 15
Clean Manufacturing & Advanced Materials
SU 262 C | 8:15 – 12:50
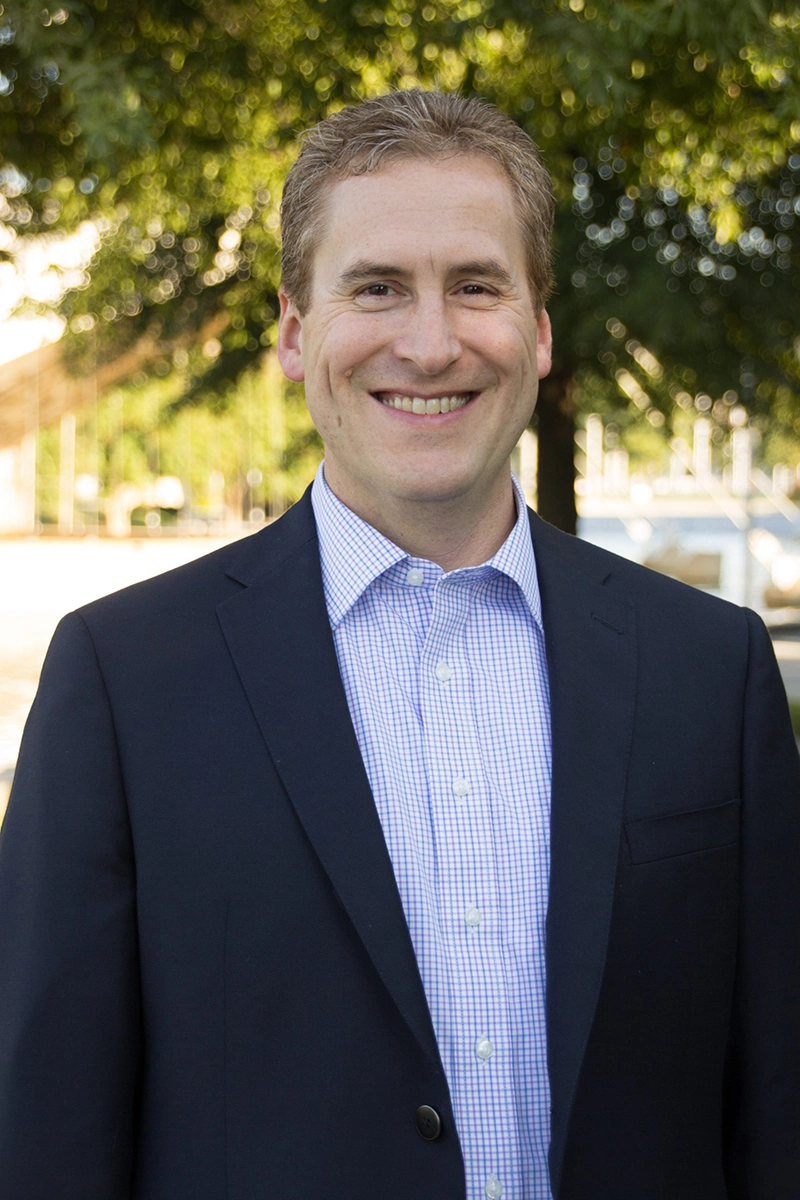
MIKE KILBEY
Molecular Design of Polymeric Materials for Energy, Sustainability, and Additive Manufacturing
Abstract
Polymeric materials are the solution to numerous technological challenges because their properties and functionality can be widely tuned through the choice and arrangement of constituent monomer units. In this presentation, I will describe our efforts to elucidate design-structure-property relationships of high performance polymers suited for advanced manufacturing, capture of carbon dioxide, and printed electronics. While each vein of research relies on a different class of advanced polymeric materials, each benefits from a molecular engineering approach to understand and expand materials-property space. For example, our work demonstrates how the character and strength of intermolecular interactions and copolymerization strategies can be used to enhance melt processibility of vinyl-addition polynorbornenes, and how chain flexibility and expansion of conjugation can improve conductivity of solution processed polythiophenes. In addition to benefitting from precision monomer and polymer syntheses, these efforts are augmented by advanced characterizations and modeling and simulation efforts that accelerate discovery and drive innovation.
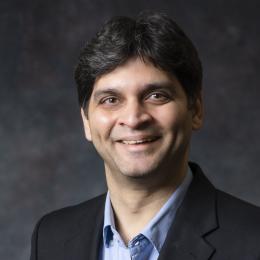
Jaydeep Karandikar
AI-informed machining
Abstract
This presentation describes the application of machine learning, a subset of artificial intelligence, to machining operations. It demonstrates the use of physics-based models to establish the initial beliefs before testing to determine the probability of milling stability (or prior). Due to model uncertainty, limited testing is completed to improve the model by Bayesian inference. Test results are shown to identify optimal stable machining conditions using an expected improvement in material removal rate criterion. The prior probability of stability is updated using the test results to determine the posterior probability of stability. Experimental results are included to demonstrate the method for industrially relevant applications
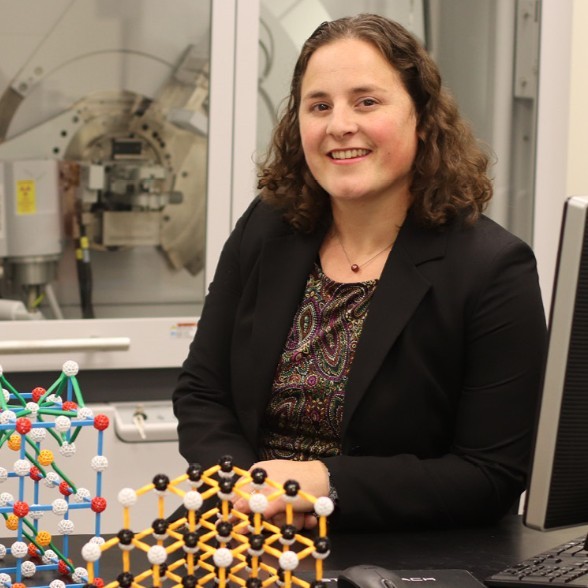
KATHARINE PAGE
Tuning Compositional Complexity in Layered High Entropy Oxides and Heterostructure Derivatives
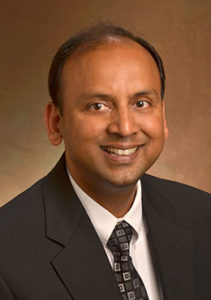
DAYAKAR PENUMADU
Innovative low cost carbon fiber based carbon-carbon composites for extreme environments
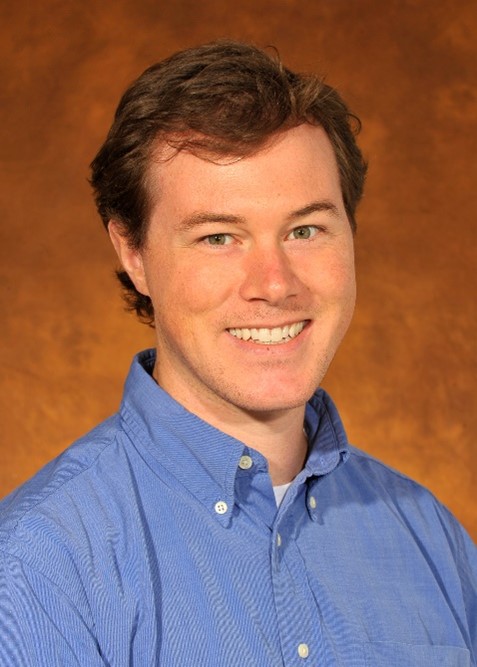
BRETT COMPTON
Additive manufacturing of composite and hybrid materials
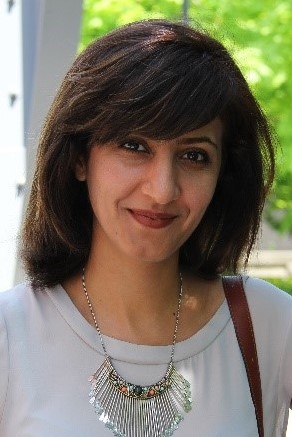
MAHSHID AHMADI
High Throughput Automated Synthesis and Characterization of Materials for Solar Cells and Other Optoelectronic Applications
Abstract
Metal halide perovskites have garnered considerable attention in the field of optoelectronics due to their exceptional properties. However, so far little has been understood based on the fundamental principles for designing the functional perovskites, which is now crucially decelerating the lab-to-fab transformation and realization of the scalable manufacturing of these materials for optoelectronics. In this talk I will discuss the potential of machine learning-driven high throughput automated experiments to expedite the discovery of hybrid perovskites, optimize processing pathways, and enhance the understanding of formation kinetics. I will showcase how high throughput automated synthesis provides a comprehensive guide for designing optimal precursor stoichiometry to achieve functional quasi-2D perovskite phases in films capable of realizing high-performance optoelectronics. I further introduce the concept of co navigation of theory and experiment spaces to accelerate discovery and design of hybrid perovskites. These studies exemplify how a high-throughput automated experimental workflow effectively expedites discoveries and processing optimizations in complex materials systems with multiple functionalities, facilitating their realization in scalable optoelectronic manufacturing processes.
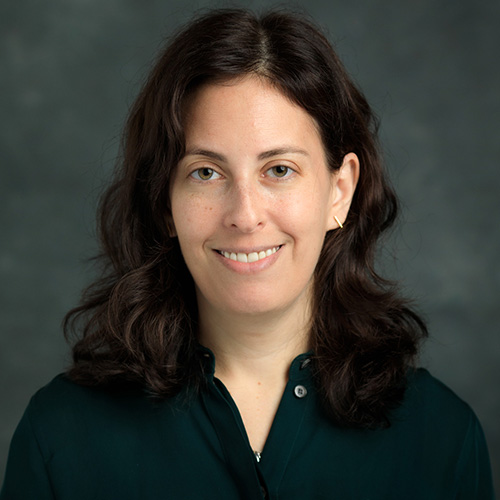
GILA STEIN
Enhancing Ionic Conductivity in Block Copolymer Electrolytes
Abstract
Lamellar block copolymers based on polymeric ionic liquids (PILs) combine the electrochemical performance of a PIL with the mechanical stability of a nonionic glassy polymer. These materials show promise as “safe” electrolytes in electrochemical devices. However, a key challenge is that the ionic conductivity of the lamellar phase is more than an order of magnitude lower than that of the PIL homopolymer. With support from the StART award, we examined three potential sources of this depressed ionic conductivity: First, the glass transition temperature of the PIL block could be elevated when linked to the glassy nonionic block, thereby suppressing ion mobility. Second, the width of the interface between the two blocks could be broad, which effectively dilutes the PIL with a nonionic material. Finally, mesoscale defects such as grain boundaries could reduce the available pathways for ion transport. We synthesized a library of PIL-based block copolymers with varying compositions and molecular weights. Each material was characterized by differential scanning calorimetry (DSC), small angle X-ray scattering (SAXS), and broadband dielectric spectroscopy (BDS), which probe the glass transition temperatures of each block, the self-assembled morphology, and the ionic conductivity, respectively. The DSC data confirm that changes in the glass transition temperature are a source of the depressed ionic conductivity, and trends with molecular weight suggest that interfacial width could also play a role. However, the trends with composition reveal that mesoscale defects are the dominant factor. Consequently, to improve the electrochemical performance of PIL-based lamellar block copolymers, efforts should focus on new materials and processing routes that tailor the transport pathways.
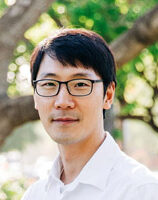
JOON SUE LEE
Topological Quantum Materials Prepared by Epitaxy
Abstract
Topological quantum materials represent a class of substances characterized by unique electronic properties due to nontrivial topology in their electronic band structures. These materials give rise to exotic electronic states and quantum phenomena at surfaces and edges, which remain robust against certain types of disorder and perturbations. Consequently, they hold significant promise for diverse applications, including quantum computing, spintronics, photonics, and sensing technologies. In this project, we focus on synthesizing high-quality thin films and nanocrystals of topological quantum materials using molecular beam epitaxy, which provides precise control over parameters such as stoichiometry, doping, thickness, and strain. Utilizing this approach, we have successfully prepared materials including alpha-tin (Sn) and BiSb and have investigated topological phase transitions and the emergence of associated topological bound states. Additionally, we have developed topological superconductors such as superconductor-semiconductor hybrid systems and FeTeSe films. This work represents a crucial step towards bridging the gap between fundamental physics and practical applications, paving the way for the development of novel technologies grounded in the unique properties of topological quantum materials.
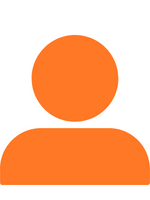
ALAN TENNANT
Materials Research Science and Engineering Center (MRSEC)
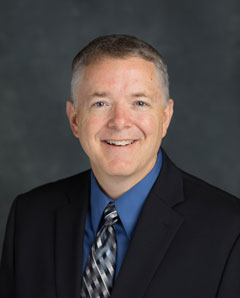
CHAD DUTY
IACMI: Developing clean energy solutions and catalyzing manufacturing competitiveness across the U.S. advanced composite ecosystem
Abstract
Headquartered in Knoxville, Tennessee, IACMI-The Composites Institute is one of six Clean Energy Manufacturing Innovation Institutes established by the Department of Energy (DOE) and supported by the Advanced Materials and Manufacturing Technologies Office. Leveraging the collective expertise of 150 members from industry, universities, national laboratories, and federal, state, and local government agencies, IACMI plays a major role in developing clean energy solutions and catalyzing manufacturing competitiveness across the U.S. advanced composite ecosystem. In addition, IACMI cultivates best-in-industry workforce development programs with partners like DOE and the Department of Defense that scale up and connect regional programs to meet the national manufacturing agenda.
Circular Bioeconomy & Decarbonization
SU 262 C | 2:50 – 4:05
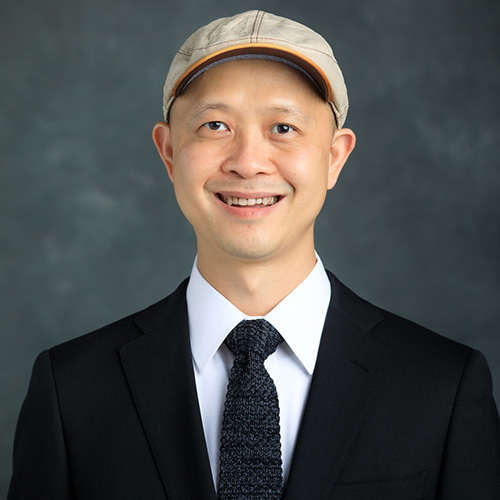
CONG TRINH
Hybrid Catalytic and Biocatalytic Processing Platform for Valorizing Mixed Plastic Wastes
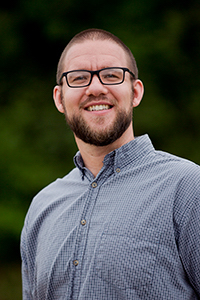
HUGH MEDAL
Protecting Power Grids From Extreme Disruptive Events
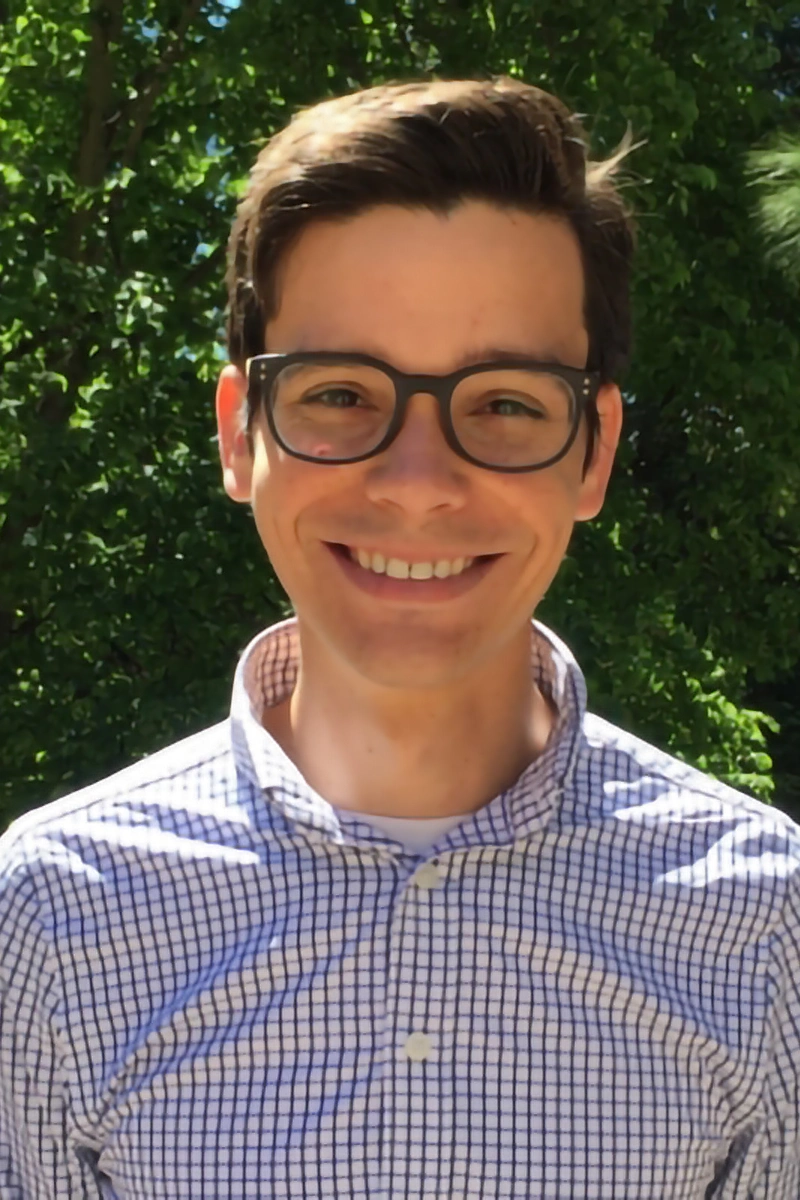
JOHN BRANTLEY
Single Electron Editing Strategies for Polymer Modification
Abstract
Accessing the chemistry of reactive intermediates under mild conditions has significantly expanded the available chemical space for molecular transformations. Photoredox catalysis and electrosynthesis are two areas where this fact is particularly true. Despite established precedent for using these methods to build complex small molecule targets, they are underutilized in macromolecular editing. Here, we describe how these tools can enable both the functionalization and degradation of polyalkenamers into valuable feedstocks. Irradiation with visible light (including natural sunlight) in the presence of a pyrillium photoredox catalyst promoted facile chain scission in a variety of substrates. Similarly, electrooxidation under mild potentials also resulted in rapid scission. Mechanistic and kinetic experiments were carried out to understand this reactivity, which could be coupled with hydrofunctionalizations (e.g., azidation, etherification, etc.) to create tailored products.
Tuesday April 16
Transportation
SU 262 C | 9:15 – 12:05
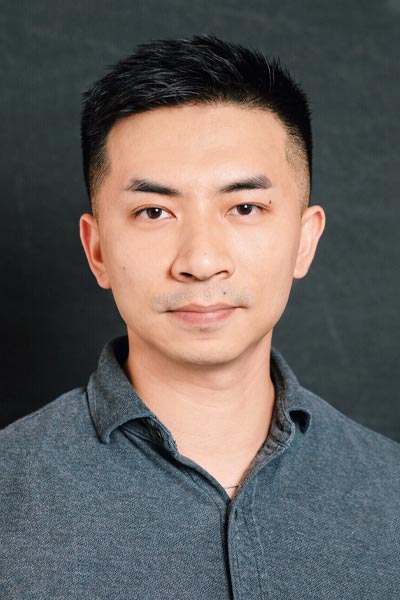
JIAN LIU
Behind Closed Doors: Exploring Privacy Vulnerabilities in Federated Learning
Abstract
The widespread adoption of Artificial Intelligence (AI) has led to a plethora of security and privacy vulnerabilities that need to be understood and mitigated. In this talk, we will explore some of the most significant privacy issues associated with AI. Specifically, the talk will examine the privacy challenges of federated learning, a collaborative machine learning approach where multiple participants train a model without sharing their data. Although federated learning is a promising technique for preserving privacy and promoting data decentralization, our recent research has revealed that private information can still be leaked through shared gradient information, even under certain defense settings (e.g., local differential privacy). By examining the emerging attack surfaces, we aim to raise public awareness about the vulnerabilities that exist in current AI-based systems, and emphasize the need for prioritizing their mitigation to prevent potential attacks.
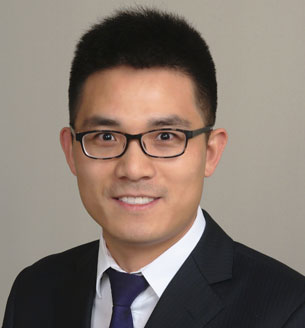
ZHENBO WANG
Enabling Safe and Efficient Transportation Systems with Connected Vehicles
Abstract
Novel connected and automated vehicle (CAV) technologies are transforming the way drivers, vehicles, and traffic infrastructure interact in real-world environments. CAVs use vehicle-to-vehicle (V2V) and vehicle-to-infrastructure (V2I) communications to coordinate traffic interaction to minimize congestion, maximize fuel efficiency, and reduce collisions. Despite the fact that the power of AI and machine learning are revolutionizing the potential impact of CAVs, much more research is required to understand how data-driven approaches interact with model-based components to better enable traffic mobility and energy savings. This two-year StART project aimed to bridge this gap by studying a novel traffic control framework intended to reconcile data-driven traffic signal phase and timing (SPaT) control and model-based speed control of CAVs. In the first part of the project, we aimed to investigate the possibility of generating optimal SPaT information using historical and real-time traffic data. State-of-the-art deep learning and reinforcement learning techniques allow us to develop scalable, adaptive, data-driven algorithms that predict optimal SPaT using extracted information from vehicular networks. In the second part of the project, we aimed to study efficient approaches to optimizing CAV movements and providing optimal speed advisories based on the generated SPaT data. Recent advances in computational optimal control and convex optimization allow us to exploit the SPaT data and develop efficient speed control strategies to improve the mobility and fuel economy of CAVs. The developed models and algorithms were demonstrated via simulations using the Simulation of Urban MObility (SUMO) simulator.
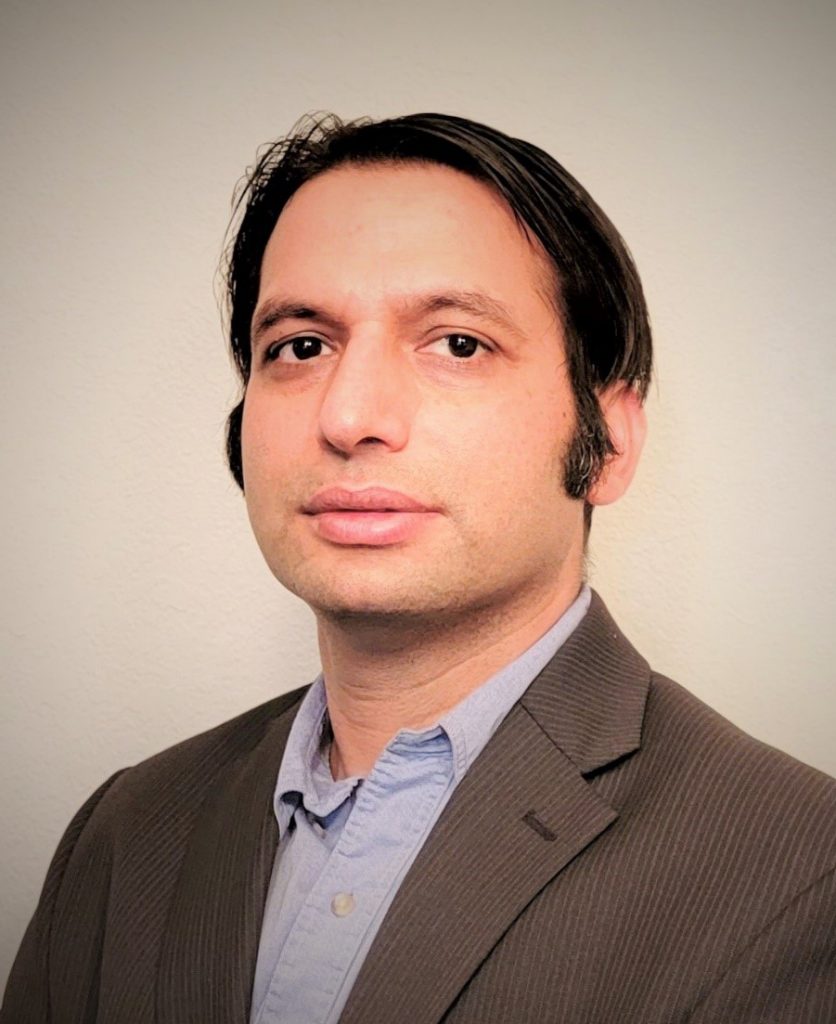
HIMANSHU THAPLIYAL
Noise-Resilient and Resource Optimized Quantum Computing
Abstract
Approximate computing represents a fresh approach to computation, aiming to yield less precise outcomes by easing the demand for absolute precision or complete determinism. In the realm of quantum computing, approximate computing offers the opportunity to compromise circuit accuracy in favor of optimizing parameters like noise fidelity, quantum depth, and resource utilization. This strategy proves especially advantageous in quantum computing application domains where exact results are unnecessary, such as machine learning, signal processing, and image processing. This presentation will delve into the realm of approximate quantum arithmetic circuits, which serve as fundamental components for complex applications. Additionally, we will provide an overview of a design automation tool capable of generating approximate quantum circuits that surpass the accuracy of baseline exact quantum circuits.
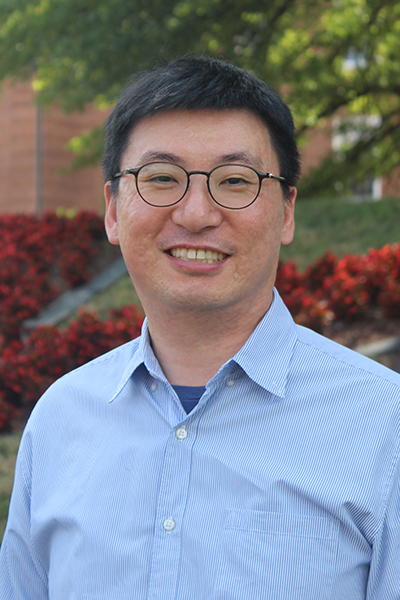
DOOWON KIM
Enhancing the Security of Connected and Automated Vehicle (CAV) Ecosystem
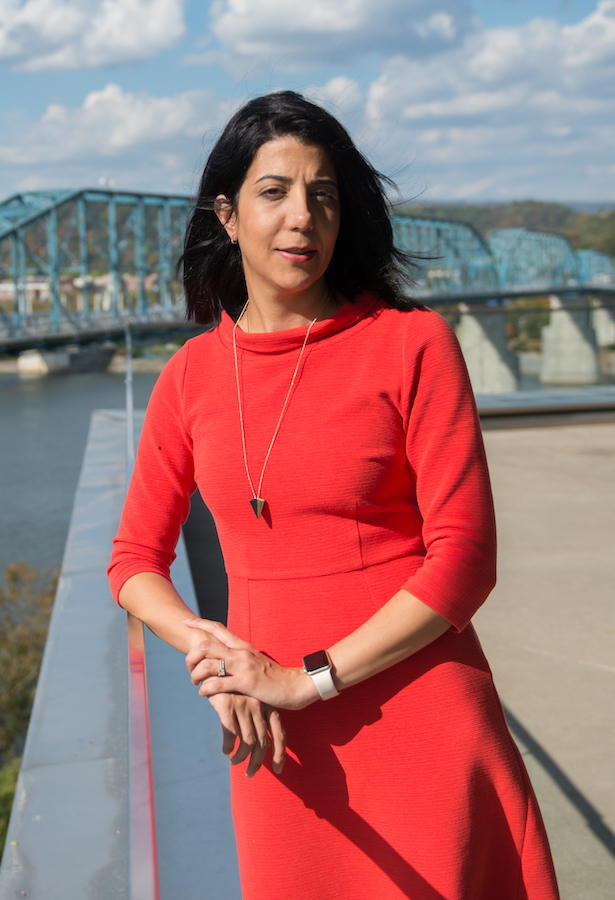
MINA SARTIPI
Smart City: Connectivity and Automation
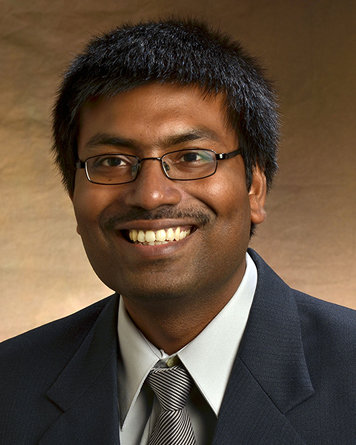
SUBHADEEP CHAKRABORTY
Driver impairment detection and safety enhancement through unified analysis of Driver, Vehicle and Traffic Volatilities
Abstract
Vehicles are generating more data than ever, and this study seeks to find a way to leverage rich vehicle kinematics data in combination with driver biometrics and information about the surrounding traffic environment to create a tool for reliable estimation of the driver’s state of distraction while operating a vehicle. The tests were conducted in a safe and immersive virtual reality environment where participants driving a simulated vehicle (using a head-mounted-display and natural controls, such as accelerator, brake pedals and steering wheel) were visually distracted by an increasingly complex task. The visual distraction was meant to emulate use of cell phones or operating a touchscreen interface and achieved by having the driver repeatedly find the randomized location of a differently-oriented arrow in a grid of otherwise similarly-directed arrows. Environmental data (such as relative distance and relative speed of traffic around the ego vehicle, deviation from the lane center-line), vehicle kinematics data, (such as speed, acceleration, steering input, accelerator and brake effort) as well as driver-biometric data (such as gaze direction, galvanic skin response, pulse rate) were collected in real time and synchronized. This combined time-series data was then used to train a multivariate time series feature extractor (WEASEL+MUSE) and a logistic regression classifier. Relative importance of the features in the dataset was examined by permuting through and quantifying the classification accuracy of combinations of features. Most importantly, the data shows that by combining the different modalities, a higher predictive accuracy can be attained than when using any one modality.
General Session
SU 262 C | 2:10 – 3:50
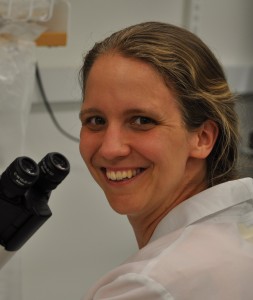
RACHEL PATTON MCCORD
Using 3D Chromosome Folding to Predict and Modify Cellular Sensitivity to Radiation
Abstract
Radiation that damages DNA can be both dangerous to healthy tissues and used as a cancer therapeutic. To develop therapeutics that destroy cancer while minimizing damage to surrounding tissues, it is critical to understand, and even manipulate, how different cell types respond to radiation. A cell’s sensitivity to radiation is in part affected by how the DNA is arranged in 3D inside the nucleus. In the first year of our StART grant, we have combined computational models (ORNL) with experimental work (UTK) to investigate how differences in chromosome folding influence the amount of damage caused by radiation in different cell types. To investigate the influence of the initial 3D chromosome folding state on radiation damage, we have pretreated healthy and cancerous epithelial cells (MCF-10A vs. MCF-7) with a drug (histone deacetylase inhibitor, TSA) that decondenses chromosome structure. The drug by itself caused no significant cellular toxicity in either cell line and did not induce any DNA damage. However, pre-treatment with this drug causes a radiosensitizing effect: cells experience more DNA damage when exposed to X-rays after TSA treatment than without this pre-treatment. This effect is more pronounced in cancer cells vs. the healthy cells, consistent with a higher initial level of open, acetylated chromatin in MCF-7 cells after TSA treatment. Our future work will measure the long term impact of this combined treatment, as well as additional chromatin structure disrupting treatments with X-rays, on cell survival, and compare these results with computational models. We will also compare the impact of X-rays with alpha particles (ORNL), which will be used in future targeted radiotherapeutics.
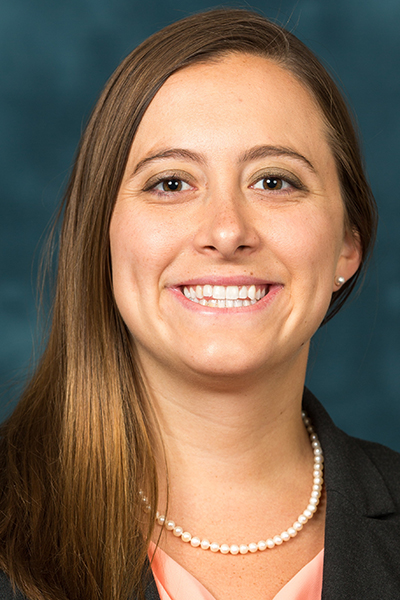
COLLEEN CROUCH
Integrated Multilayered Spatial Analysis of Age, Sex and Alzheimer’s Induced Changes In Brain Biochemistry
Abstract
Alzheimer’s Disease (AD) is a complex neurodegenerative disorder in which the underlying mechanisms for disease initiation and progression are not understood. Researchers have identified two hallmarks of AD, neurofibrillary tangles of hyperphosphorylated tau and amyloid plaques of amyloid-β (Aβ), yet there are no effective FDA-approved treatments. Importantly, plaques occur in elderly patients without AD diagnosis, and elderly patients without plaques can have impaired cognition. This underscores the need for a basic science approach to understanding the molecular mechanisms of AD and integrating molecular studies with observable cognitive deficits. Additionally, while the risk and prevalence of AD increase with age and two-thirds of patients with AD are women, the impact of age and sex on brain biochemistry remains unclear.Without a fundamental understanding of the intersectional effects of age, sex and genotype, an effective treatment for AD and other dementia-related neurological diseases will remain elusive. The overall goal is to use a multimodal protocol with explainable-AI to map phenotypic signatures of the brain with observed cognitive deficits to identify molecular changes due to aging, sex, or genetics. We expect to uncover molecular changes not previously associated with AD, aging, or sex. We will use computational algorithms to integrate data from cognitive testing followed by mass spectrometry imaging, liquid chromatography coupled with ion mobility spectrometry mass spectrometry of aggregated cells, and spatial RNA sequencing of brain sections to create a 2D molecular map. We have found significant differences in lipids in aged vs. young mouse brains across genotypes. We propose to fill these critical gaps in fundamental knowledge by taking an unbiased molecular approach to investigating the spatial distribution of brain molecules combined with translationally-relevant behavioral indicators of cognitive decline. This approach will provide new information on the initiation and progression of biomolecular changes across brain regions underlying cognitive decline in AD.
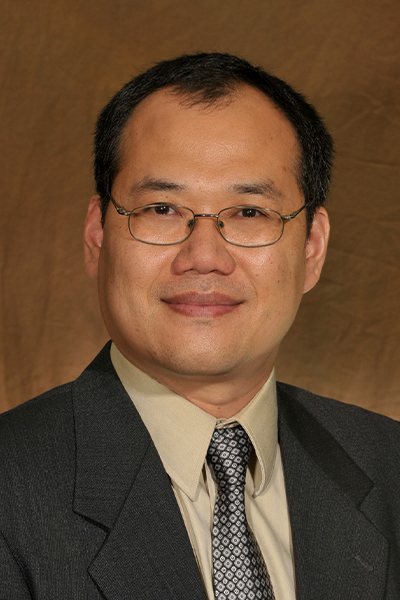
XIAOPENG ZHAO
Collaborative AI and Robotics to Empower Persons with Dementia (CARE4PwD)
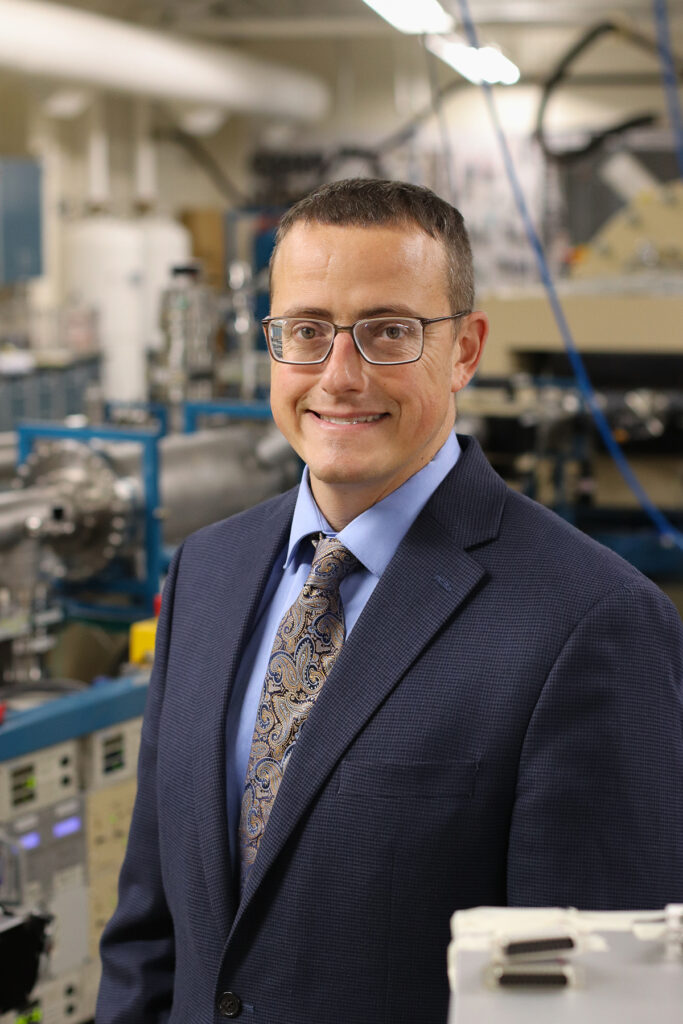
KHALID HATTAR
Coupling Extreme Environments at the Tennessee Ion Beam Materials Laboratory
Abstract
A fundamental understanding of materials response to coupled extreme environments is necessary for everything from long term manned interstellar missions to the design and production of advanced fusion and fission energy systems. The Tennessee Ion Beam Materials Laboratory (TIBML) is a University of Tennessee core facility that has undergone a rapid evolution over the last year. TIBML has a long and rich history as a world-class Ion Beam Analysis (IBA) facility for elemental analysis of surface and subsurface regions. Over the last year, the facility has undergone a rapid growth phase to expand the ability to explore the response of materials to coupled extreme environments. These environments include ion irradiation, ion implantation, heating, cryogenic cooling, and soon mechanical testing. Work is underway to couple these extreme environments. This presentation will highlight the evolution of the facility, detail the current capabilities, and discuss the potential applications in materials ranging from biological samples through quantum devices to additive manufactured refractory high entropy alloys. The presentation will end with how ion beam modification can be used for tailored far-from-equilibrium nanoscale structures, as will be demonstrated with kryptonite as an exemplar.
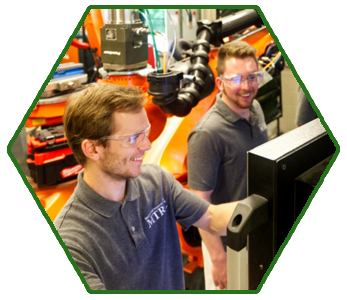
Student poster presentations highlight the meritorious and collaborative research conducted by graduate students funded through the Graduate Advancement, Training and Education (GATE) program and faculty-led UT-ORII grants.