Support for Affiliated Research Teams (StART)
In FY20 the Science Alliance introduced the Support for Affiliated Research Teams (StART) program. StART is designed to deepen the collaborative spirit between the University of Tennessee and Oak Ridge National Laboratory. The program issues an annual call for proposals with two subsequent submission deadlines. Proposals are evaluated on technical merit, potential for continuing and meaningful collaborations, inclusion of graduate and undergraduate students, and the likelihood for future external funding. The first awards were announced in August, 2019.
Since the program started, 13 joint research projects have been funded, totaling more than $1.4 million. The StART program differs from other UT-ORII funding opportunities in that it is the primary mechanism for funding nascent research teams and gives preference to investigators that are establishing their first collaboration between the institutions.
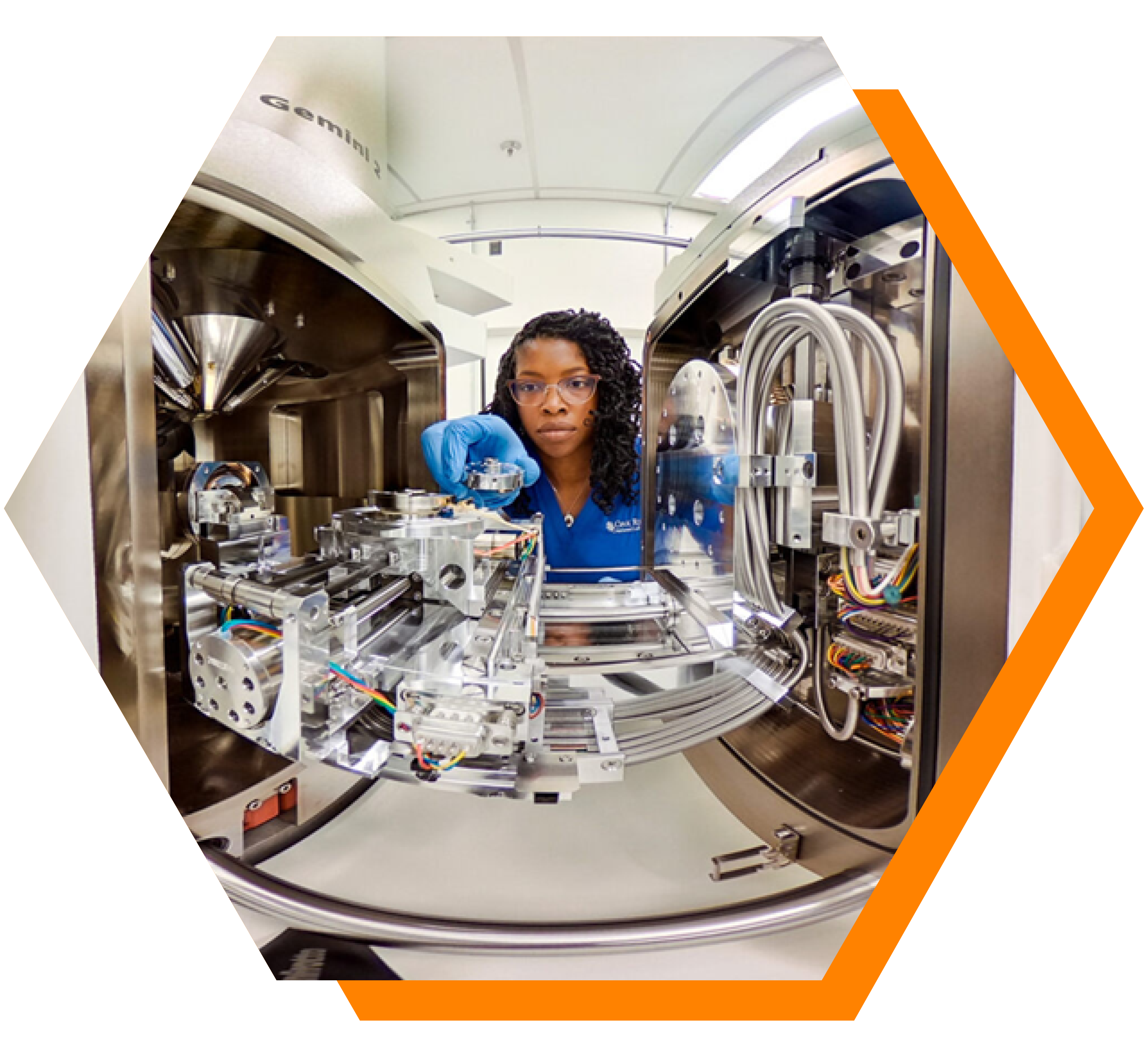
Applying for StART
StART has a single call, with semiannual deadlines. Typical awards are $100k. The number of awards is dependent on the quality of proposals submitted, as well as available funds. Projects will be funded for one year, with a chance for second-year funding if the project shows strong promise of receiving new external funding as a result of the collaborative activities leading to preparation of a joint proposal for external funding that is deemed meritorious in the second year’s proposal evaluation process.
The deadline for FY 25 proposals was May 15, 2024. Award notifications will be sent out after June 30, 2024.
Eligibility
StART was established to foster collaboration between UT and ORNL researchers. Therefore, each research team must include a principal investigator from UT and confirmed collaboration with an ORNL scientist. All UT faculty members are eligible to apply, including faculty who have had previous ORNL collaborations and/or have existing joint appointments. Post-docs, research scientists, and lecturers are ineligible to apply for this program. Preference will be given to junior faculty who do not have a joint appointment and/or are establishing a new collaboration with ORNL scientists.
Expectations of Awardees
A primary focus of the StART program is to generate new funding for collaborative teams of scientists and engineers. Therefore, each funded StART team will be expected to have either submitted a new external proposal by the end of the first year or to provide the Science Alliance with a draft proposal that will be submitted within three months of the end of the performance period for the award. This proposal must include support for all UT and ORNL principal investigators. Second-year funding will only be available to teams that provide the above mentioned proposal to the Science Alliance team. These proposals will count as the proposal submission for year two internal funding as well.
Review Process and Criteria
A review committee appointed by the director of Science Alliance will review the proposals and make recommendations for funding. Proposals will be evaluated based on the following criteria:
- Technical merit of the proposed research
- Potential for the establishment of a meaningful and sustainable collaboration with ORNL, including joint publications, student mentoring, team development, etc.
- Inclusion of graduate students in the research
- Likelihood for future external funding and target funding call/notice
First Year Application Instructions
A complete year-one application will include the following:
A. Research Proposal (3-page max, add additional pages for references)
- Project summary written for a general audience (150-word max)
- Description of the proposed research
- Description of specific funding opportunities that will be targeted for future support inclusive of funding agency, program manager/officer and prior interactions with funder
- Description of all of your prior affiliations, collaborations, and publications with ORNL, and how this project represents a new interaction in a new scientific/engineering area
- Description of expected extended collaborations with your ORNL colleagues, for example: joint faculty opportunities, student mentoring, team development, publications,etc.
B. Appendices
- Appendix A: Budget and budget justification* (template available via InfoReady listed under the Competition file section – top right side)
- Appendix B: A letter from the ORNL PI supporting the partnership and outlining the level of commitment
- Appendix C: A one-page bio for each UT/ORNL PI that includes most recent grants, professional awards, and recent relevant publications
Second Year Application Instructions
A complete second-year renewal application will include the following:
A. Research Proposal Prepared for External Funding Agency (format will vary based on target agency)
B. Appendices
- Appendix A: Budget and budget justification* (template available via InfoReady listed under the Competition file section – top right side)
- Appendix B: An endorsement letter from the ORNL investigator stating the specific impact of the previous year’s partnership and their desire to continue with the partnership
- Appendix C: A one-page bio for each PI that includes most recent grants, professional awards, and recent relevant publications of both the UT and ORNL investigators
* Budget Allowable Costs
Allowable costs include:
- Graduate student support
- Undergraduate student support
- Postdoc support
- Supplies
- Other incidentals to support the research
- Academic year and/or summer salary (applicable to all colleges) allowed up to a maximum of $5,000 per year, inclusive of benefits
- Benefits for all personnel
- Individuals’ accrued benefits before participating in the StART program with a department head letter covering accrued annual leave
Unallowable costs include:
- Membership fees
- Conference registrations
- Equipment is discouraged and is unlikely to be awarded.
- Travel – except where travel is necessary to meet with ORNL collaborators or external funding agencies and/or program
- Expenditures above 5% outside the budget categories require approval from the Science Alliance director.
- F&A charged to the project
IMPORTANT: If the project is selected for funding, the funds must be used as requested in your project budget unless prior approval is granted, and unused funds will be reclaimed at the end of the project year.
Please contact Amber Mathes at acolbur1@utk.edu if you have any questions about the application procedure.
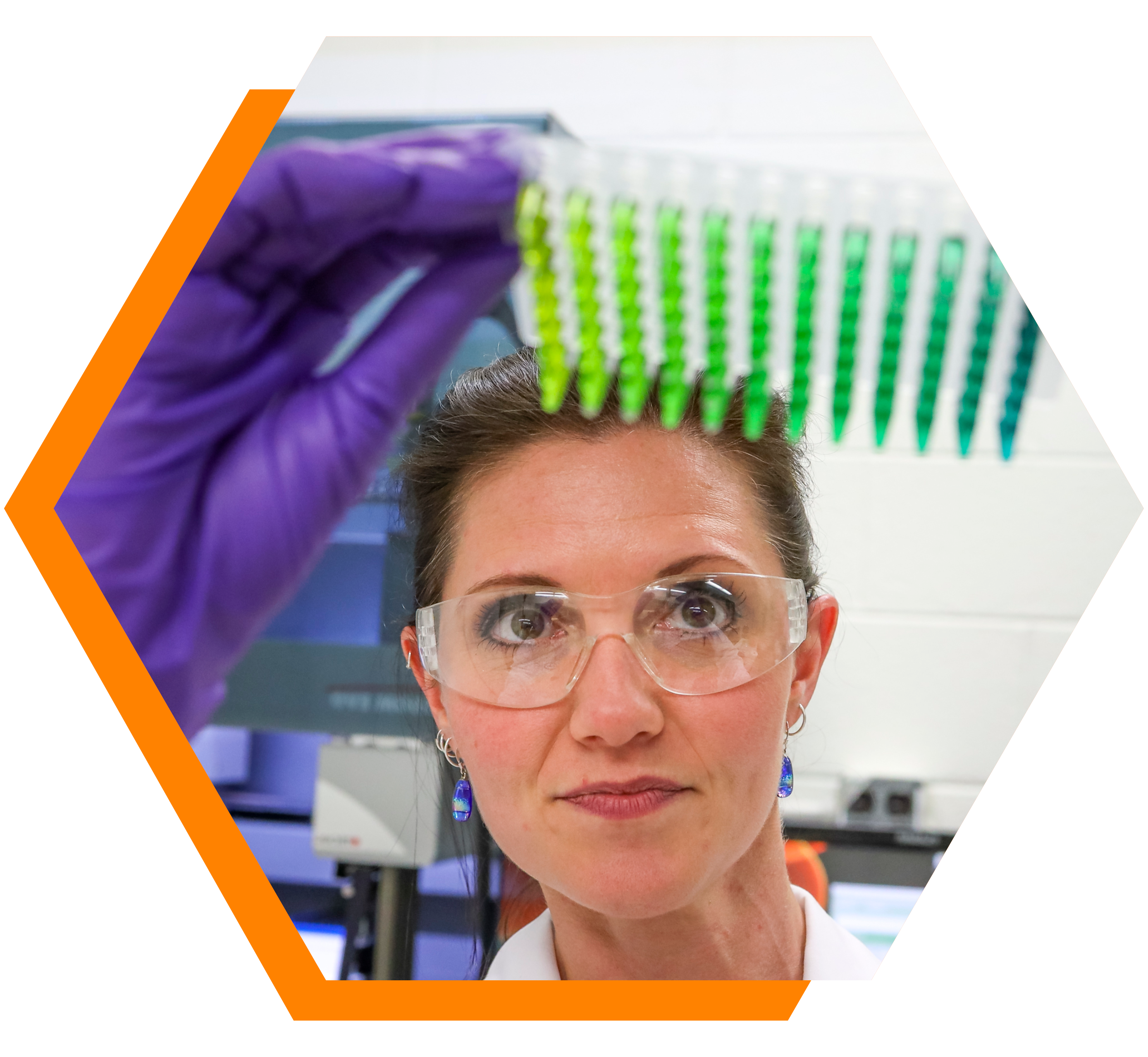
The StART program has funded 20 research teams representing over $2 million in joint UT-ORNL research investment since 2019.
Current StART Awardees
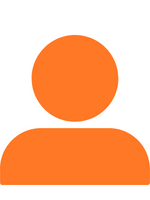
Coming Soon
Department
Coming Soon
2023 – 2024 Awardees
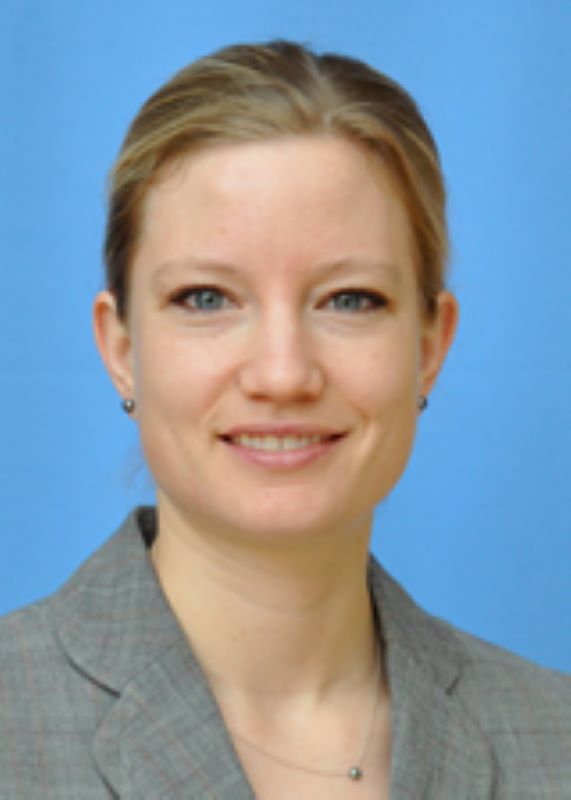
Jennifer DeBruyn
Biosystems Engineering & Soil Science
Microbial cascades from animal decomposition hot spots in Sphagnum bogs
Understanding ecosystem carbon and nutrient flows is critical to predicting responses to a changing climate. Sphagnum-dominated bogs (peatlands), which currently store >25% of global terrestrial carbon, are under threat of becoming a source of greenhouse gasses as rising temperatures increase microbial decomposition of long-stored organic matter. Quantifying microbial decomposition is thus essential to future climate models. Our objective is to determine how decomposing animals alter microbial communities and their nutrient and carbon cycling activities inSphagnum bogs.
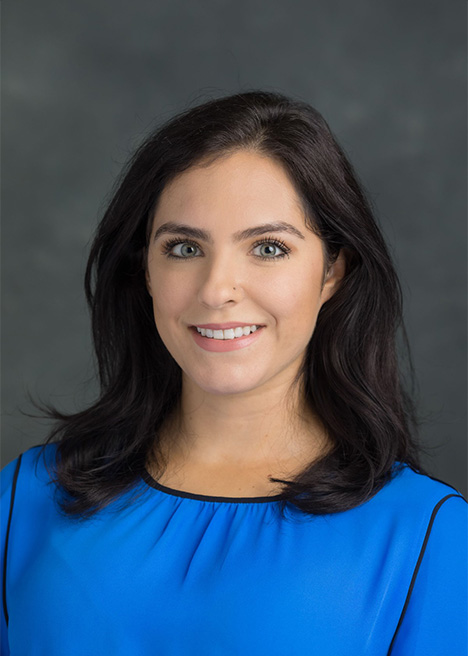
Ivis Chaple Gore
Development of Novel Diagnostic Radiopharmaceuticals for Early Detection of SSTR+ Neuroendocrine Tumors
Neuroendocrine tumors (NETs) are a rare type of vascularized tumor with a prevalence of ~170,000 patients in the United States, with diagnoses increasing each year. Survival rate for patients with NETs varies by extent and location of disease, with pancreatic NETs having the highest risk of death and a five-year survival rate of ~22.7%. There are only a handful of FDA approved radiopharmaceuticals that can be used to diagnose and/or treat NETs. Nuclear medicine is a great tool for diagnosis of NETs because it enables us to accurately and non-invasively assess disease with a single scan. The radiopharmaceuticals we propose to develop will aid in the diagnosis of NETs.
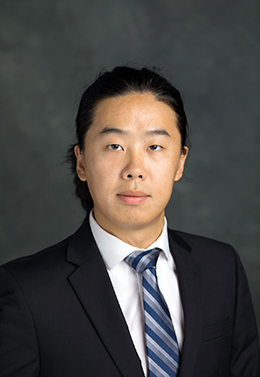
Haochen Li
Civil & Environmental Engineering
AI-empowered bio-inspired flexible turbine for efficient wave energy harvesting
Wave energy could serve as a reliable, low-cost, and zero-carbon energy source. However, existing wave energy harvesting systems such as oscillating water column Wells turbines have limited deployment in practice. This primarily stems from the low energy conversion efficiency of such turbine systems, despite their simple construction and low maintenance cost. The energy conversion efficiency of Wells turbines is fundamentally constrained by their symmetrical shape, which converts oscillating air pressure into unidirectional rotation. Inspired by the ubiquitous example of flexible wings and fins in nature, we propose a novel solution of using biomimetic flexible turbine blades to enable passive pitch control and enhance the Wells turbine performance.
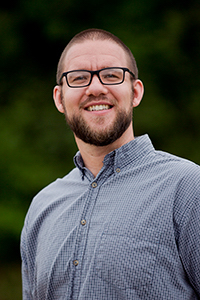
Hugh Medal
Industrial and Systems Engineering
Protecting Power Grids Using Decision-Dependent Uncertainty
To create reliable, resilient, sustainable, and equitable power systems, there is a need to develop excellent design planning tools. The overall objective of this project is to develop an interoperable and flexible MDPT built using a decision-dependent, non-linear stochastic model. This study is the first to consider redefining the boundaries of microgrids as part of the reconfiguration responses while factoring in the unique disturbances stemming from the adoption of Variable Renewable Energies. This project will develop an MDPT that incorporates the complex operational considerations of distributed energy resources and Variable Renewable Energies while considering the hierarchical nature of networked microgrids in the budding power distribution networks.
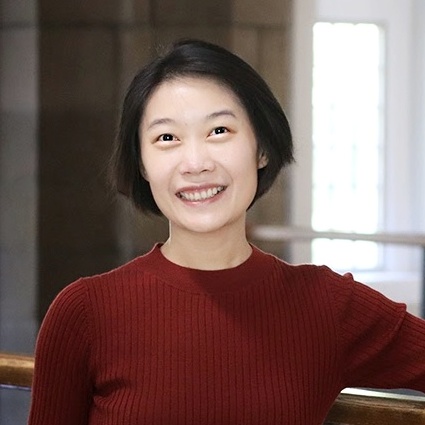
Yishu Wang
Materials Science & Engineering
In situ Neutron Reflectometric and Thermo-Magneto-Electric investigations of quantum devices
This project develops an experimental scheme for in situ neutron reflectometry to characterize the energy-harvesting thermo-magneto-electric process in quantum devices known as spintronics. The aim of this research is to address the growing potential of spintronics for information technology by providing direct insights into interface magnetism and its crucial role in device functionality. During the initial period, the project will fabricate and analyze spintronic devices at UTK and customize the neutron scattering setup at SNS’s BL4A Magnetism Reflectometer.
2022 – 2023 Awardees
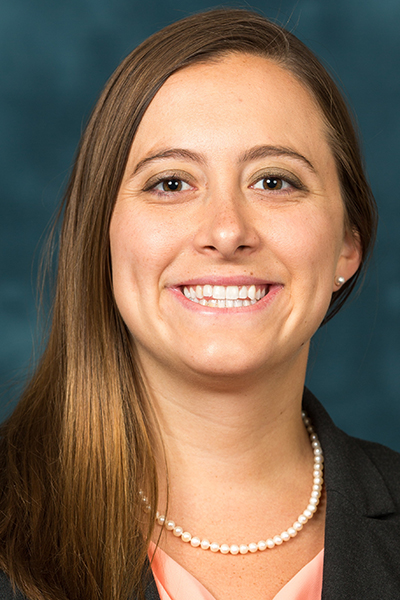
Colleen Crouch
Mechanical, Aerospace and Biomedical Engineering Department
Brain Spatial Multi-omic Imaging & Analysis Protocol: Alzheimer’s Disease Proof of Concept
Mapping phenotypic signatures of the brain can be used to identify changes due to aging or disease through development of multimodal research protocol. Better understanding of the spatial heterogeneity of phenotypic changes occurring during disease progression will allow more targeted diagnosis and treatment. Using computational algorithms to integrate data, generated from mass spectrometry imaging, microscopy-guided single cell MS profiling, and spatial single-cell RNA sequencing from tissue sections of targeted brain regions to create a 3D atlas/map of a portion of the brain. Previously, these 3 techniques have not been combined previously.
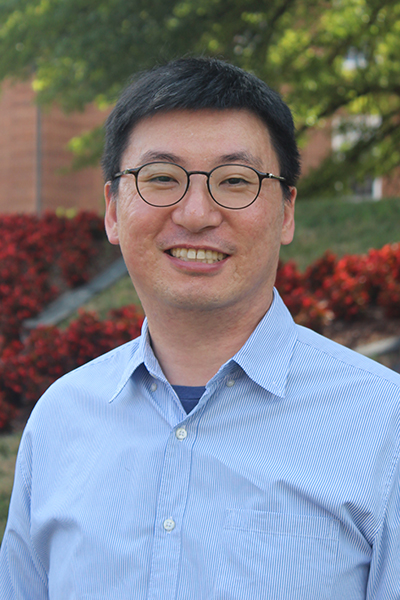
Doowon Kim
Min H. Kao Department of Electrical Engineering and Computer Science
Enhancing the Security of Connected and Automated Vehicles Ecosystem
Connected and automated vehicles (CAVs) are considered a future, intriguing technology that can change our daily lives on future roadways in terms of drivers’ safety and fuel efficiency through the communications between vehicles and infrastructures. Despite these advantages, security has not been the first priority in the CAVs ecosystem; instead, the efficiency of algorithms has always been the higher priority. Therefore, this can open up unprecedently various attack surfaces for adversaries.
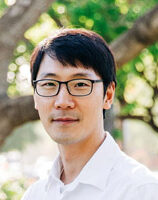
Joon Sue Lee
Department of Physics and Astronomy
Topological Quantum Materials Prepared by Epitaxy
Topology, a way of thinking about objects based on their broad properties that are preserved under continuous deformations, can be applied to condensed matter physics. In the context of quantum materials, topology can explain and predict why novel electronic states emerge at surfaces/edges and interfaces with unusual properties such as spin-charge coupling and novel superconducting features, which make the topologically nontrivial materials (topological materials) exciting candidates for future device applications.
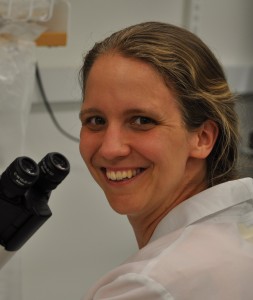
Rachel Patton McCord
Biochemistry & Cellular and Molecular Biology
Predicting and modifying cellular radiosensitivity with 3D genome folding
Radiation that damages DNA can be both dangerous to healthy tissues and used as a cancer therapeutic. To develop therapeutics that destroy cancer while minimizing damage to surrounding tissues, it is critical to understand, and even manipulate, how different cell types respond to radiation. A cell’s sensitivity to radiation is in part affected by how the DNA is arranged in 3D inside the nucleus. Iterative prediction and experiment, will reveal the relationship between 3D genome structure and cell radiosensitivity and identify structure manipulations that increase cancer susceptibility to radiation therapies.
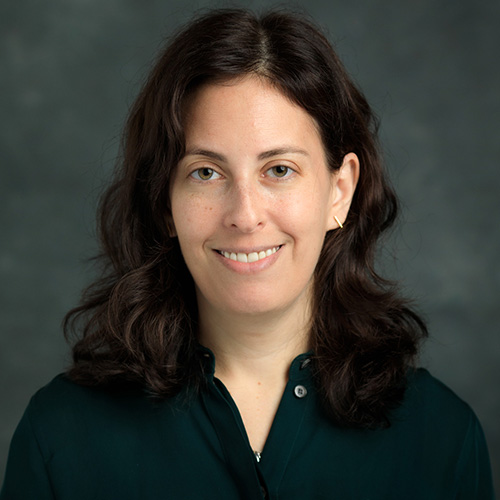
Gila Stein
Chemical and Biomolecular Engineering
Design Rules to Elevate Ionic Conductivity in Block Copolymer Electrolytes
Block copolymers comprised of a polymeric ionic liquid linked to a nonionic polymer are a class of “safe” electrolytes with tunable mechanical properties. Recent work has showed that ionic conductivity in these materials is significantly depressed relative to predicted values, posing a challenge for applications in energy storage. It’s believed his depression is a result of mesoscale defects that inhibit ion transport, local effects associated with molecular design, or a combination of these factors. Systematic structure function studies will provide a foundation to improve ionic conductivity through materials processing and/or molecular design.
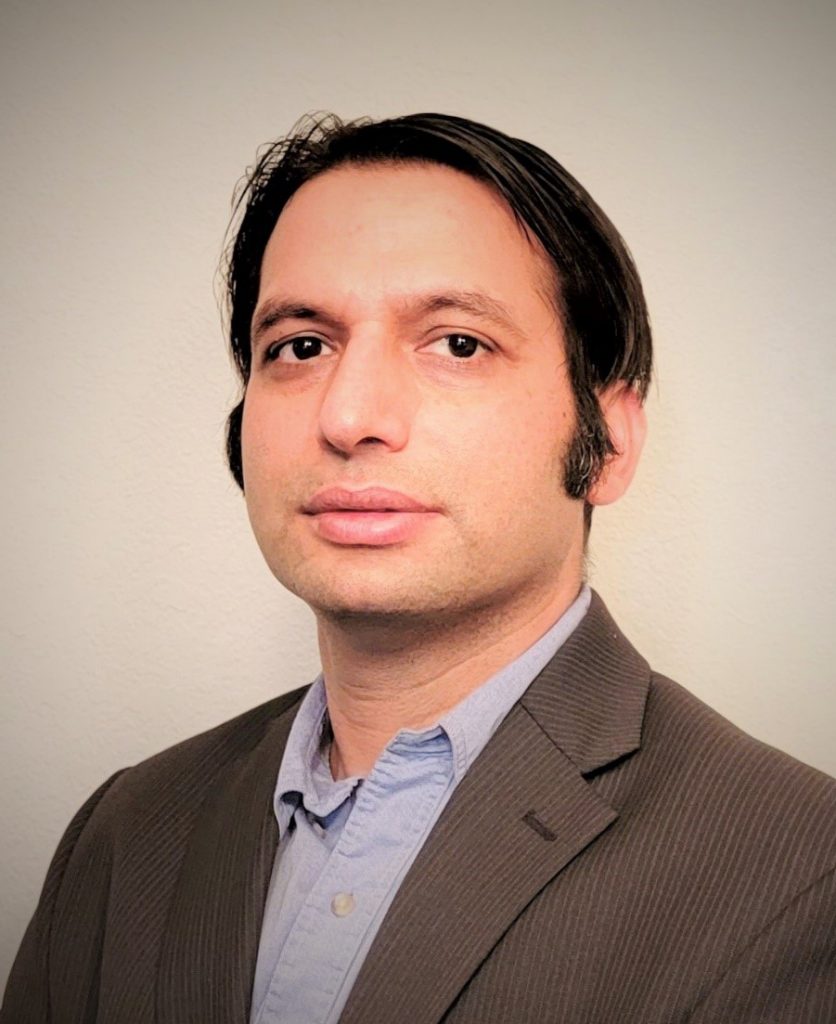
Himanshu Thapliyal
Min H. Kao Department of Electrical Engineering and Computer Science
A Cross-Layer Application of Approximate Computing to Increase Noise Resilience of NISQ Quantum Circuits
Fully fault-tolerant quantum computation will take a significant amount of resources. Therefore, one of the current focuses in quantum computation is to establish the utility of small scale, error-prone, or “noisy intermediate-scale quantum” (NISQ), machines. In NISQ machines, the application of quantum gates as well as the measurement operations can introduce errors
2021 – 2022 Awardees
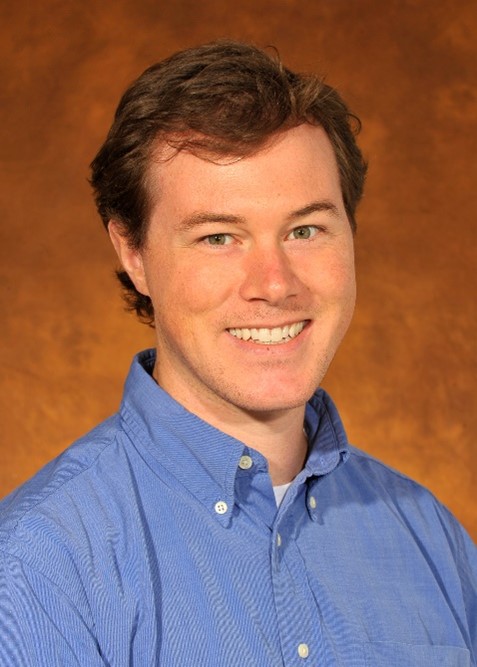
Brett Compton
Department of Mechanical, Aerospace, and Biomedical Engineering
High temperature, damage-tolerant hybrid materials through precision additive manufacturing of multi-phase architectures
Compton’s project will investigate the processing and properties of high-precision brick-and-mortar architectures composed of high temperature ceramic and carbon materials for applications in nuclear power, air- and land-based turbines, high power electronics, and more.
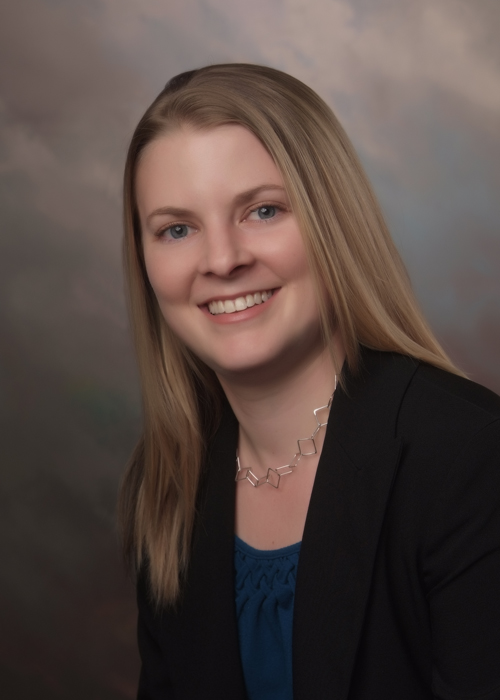
Kristina Kintziger
Prospective and Longitudinal Multivariate Study of Post-Acute SARS-COV-2 Infection Syndrome (PaLM-COVID)
Kintziger plans to conduct a prospective longitudinal study of a large group of individuals recovering from COVID-19. This study should increase scientific understanding of the COVID-19 respiratory illness and how if affects people over time.
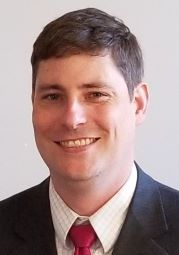
Eric Lass
Department of Materials Science and Electrical Engineering
Al-Ce Deformation Processing
Al-Ce-based eutectic alloys exhibit remarkable strength and better microstructural stability than many other alloys, but the ultrafine eutectic microstructure credited with this behavior only forms at high solidification-rates. This project will investigate the use of thermo-mechanical processing to create similar microstructures, broadening potential applications of the alloys.
Second Year Funding Awards
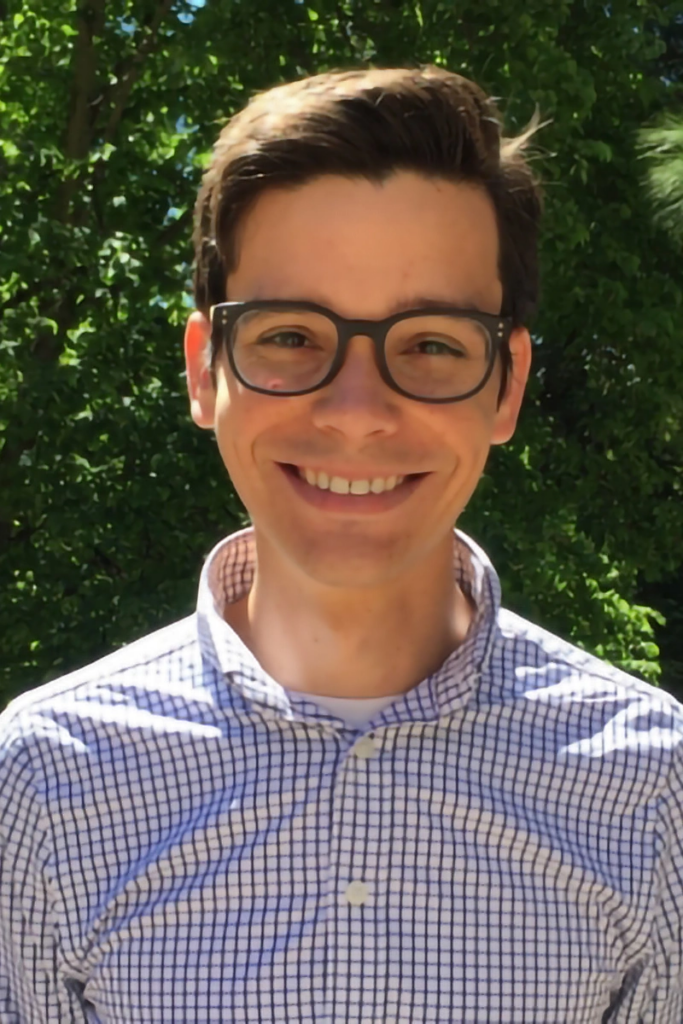
Johnathan Brantley
Cyclic Cumulenes as Enabling Motifs in Functional Materials
Polymers that contain cyclic repeating units are important synthetic targets, given they often exhibit unique physical properties. Brantley’s project will explore vinyl-addition polymerizations of cyclic allenes to access materials with new properties and potential applications.
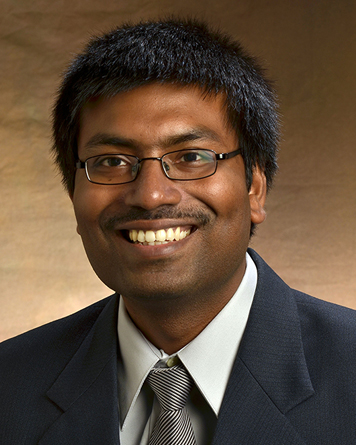
Subhadeep Chakraborty
Department of Mechanical, Aerospace and Biomedical Engineering
Artificial Intelligence based impairment detection system for vehicle operators through combined analysis of physiological and traffic sensor data
Impaired driving is a key contributing factor leading to more than 10,000 fatalities in 2016. By integrating and fusing multiple data sources such as driver biometrics, vehicle kinematics, and roadway and environmental conditions in real-time, this project aims to generate an intelligent Advanced Driver Assist System (iADAS) which will provide useful feedback to drivers and potentially mitigate accidents.
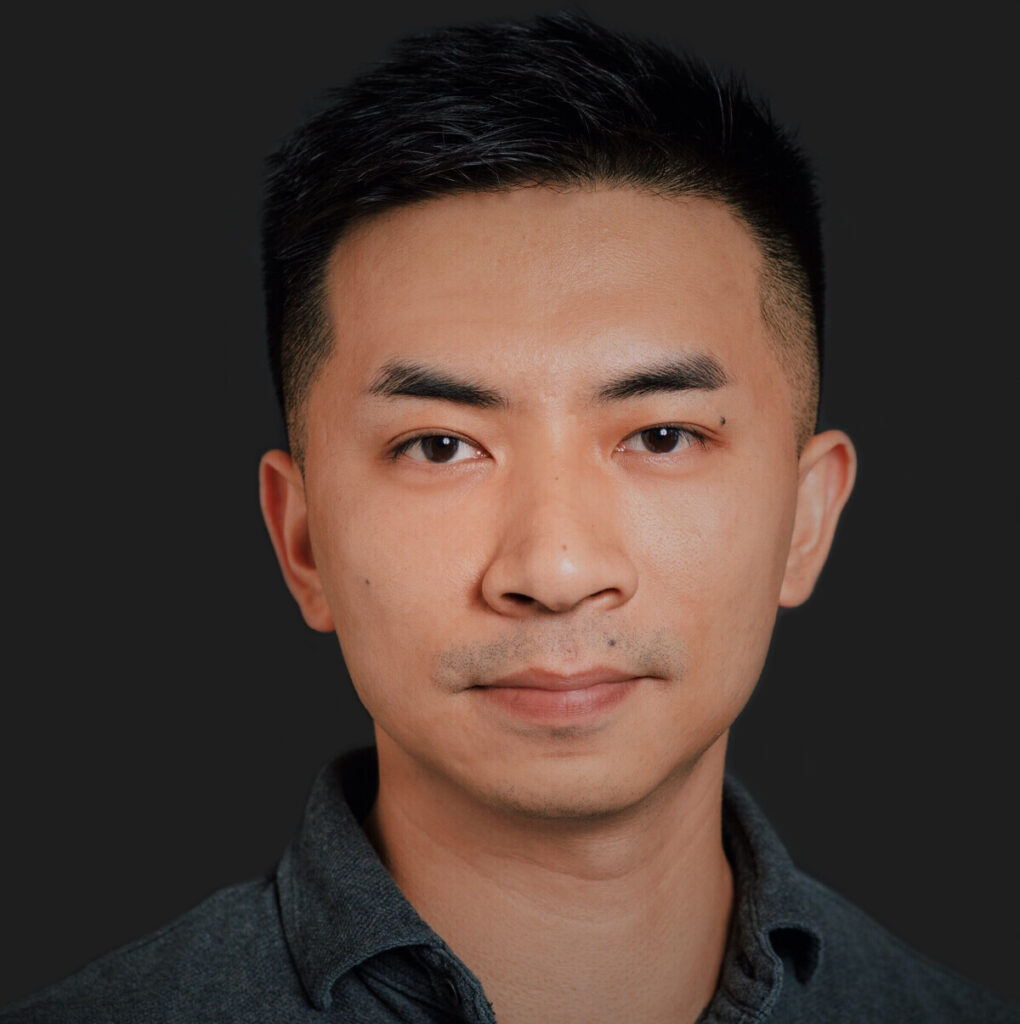
Jian Liu
Min H. Kao Department of Electrical Engineering and Computer Science
Towards Robust and Trustworthy Federated Learning for Ubiquitous Cyber-Physical Systems: Security, Privacy, and Scalability
Different from traditional centralized training, federated learning distributes the training process to the edge, enabling edge-computing devices to collaboratively learn/update a shared model using the data that is kept locally on the device. Liu’s project hopes to build a foundation for understanding how to push AI gains in performance, robustness, and scalability to CPS in mobile edge computing.
2020 – 2021 Awardees
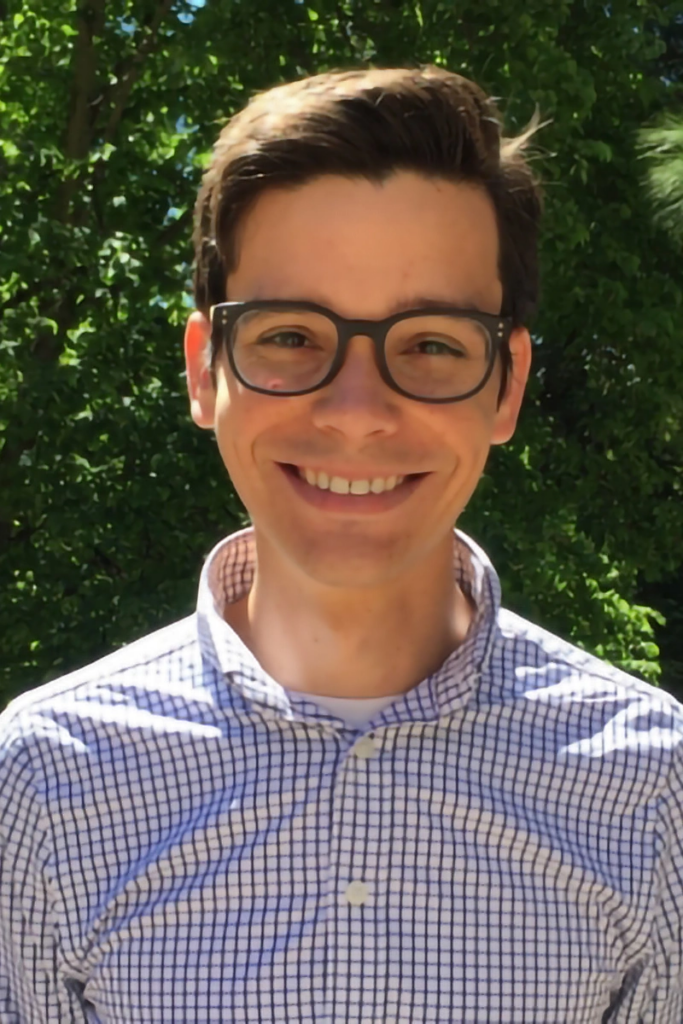
Johnathan Brantley
Cyclic Cumulenes as Enabling Motifs in Functional Materials
Polymers that contain cyclic repeating units are important synthetic targets, given they often exhibit unique physical properties. Brantley’s project will explore vinyl-addition polymerizations of cyclic allenes to access materials with new properties and potential applications.
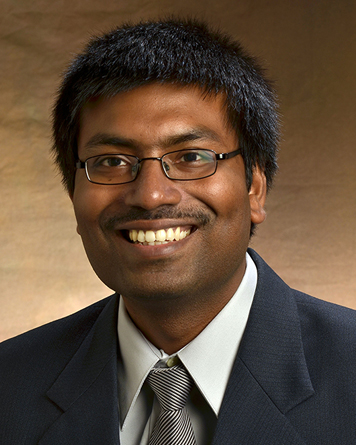
Subhadeep Chakraborty
Department of Mechanical, Aerospace and Biomedical Engineering
Artificial Intelligence based impairment detection system for vehicle operators through combined analysis of physiological and traffic sensor data
Impaired driving is a key contributing factor leading to more than 10,000 fatalities in 2016. By integrating and fusing multiple data sources such as driver biometrics, vehicle kinematics, and roadway and environmental conditions in real-time, this project aims to generate an intelligent Advanced Driver Assist System (iADAS) which will provide useful feedback to drivers and potentially mitigate accidents.
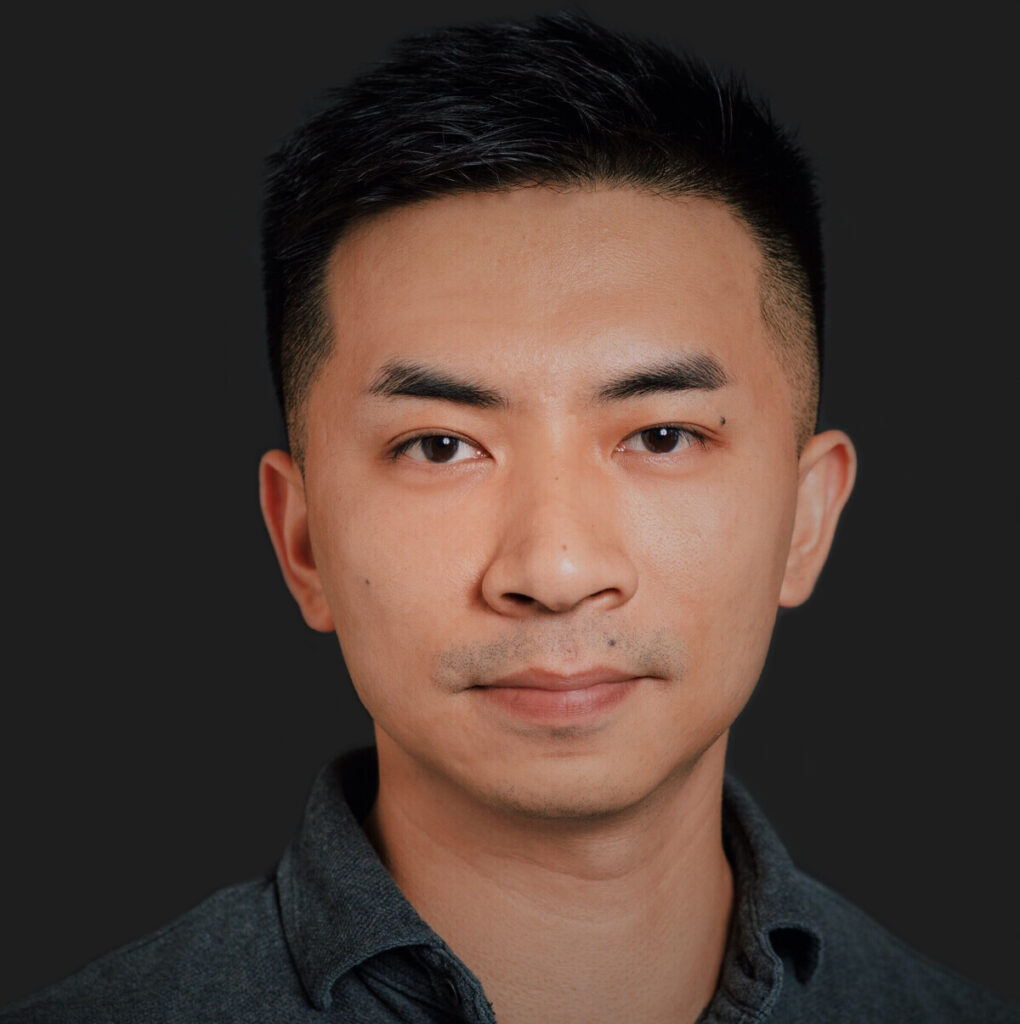
Jian Liu
Min H. Kao Department of Electrical Engineering and Computer Science
Towards Robust and Trustworthy Federated Learning for Ubiquitous Cyber-Physical Systems: Security, Privacy, and Scalability
Different from traditional centralized training, federated learning distributes the training process to the edge, enabling edge-computing devices to collaboratively learn/update a shared model using the data that is kept locally on the device. Liu’s project hopes to build a foundation for understanding how to push AI gains in performance, robustness, and scalability to CPS in mobile edge computing.
Second Year Funding Awards
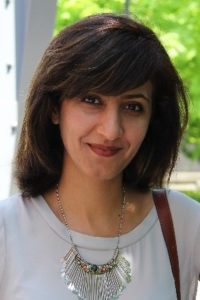
Mahshid Ahmadi
Department of Materials Science and Engineering
Machine Learning Driven Experimental Approach for the Discovery of New Organic-Inorganic Halide Perovskites for Optoelectronic Applications
Organic-inorganic halide perovskites have emerged as materials of choice for low-cost photovoltaics and optoelectronics due to relatively easy solution synthesis and unique spectrum of functional properties. The objective of this project is to establish a machine learning based experimental approach towards discovery and prediction of hybrid perovskites properties via combinatorial synthesis and evolutionary experiment optimization.
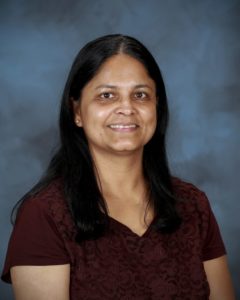
Sindhu Jagadamma
Department of Biosystems Engineering and Soil Science
Is Soil Manganese a Major Driver for Organic Carbon Cycling in Croplands?
Manganese (Mn), an essential plant micronutrient, is believed to play a critical yet poorly understood role in terrestrial ecosystem carbon (C) cycling, particularly in human manipulated agoecosystems. Her team proposes a series of field and laboratory experiments that simulate excess Mn mobilization in agricultural soils to quantitatively assess the role of Mn in ecosystem C cycling. The findings of this proposed study will inform whether acidity-induced elevated Mn availability is a critical driver in soil organic C storage/loss in highly managed agroecosystems.
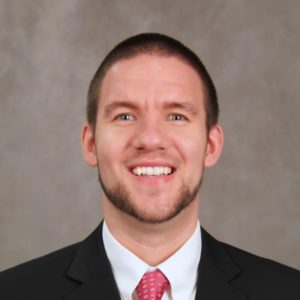
Hugh Medal
Department of Industrial and Systems Engineering
Machine-Learning-Enabled Modeling for High-Dimensional Dynamics of Materials Processing
Understanding the movement of ions through material is crucial to understanding important properties such as radiation, stress cracking, and ion conductivity. Because of this, there is an urgent need to map high-dimensional energy landscapes (HDEL). Medal’s team plans to address this need via machine-learning-enabled modeling.
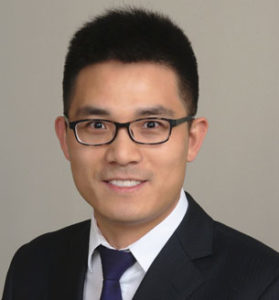
Zhenbo Wang
Department of Mechanical, Aerospace and Biomedical Engineering
Real-Time Control for Connected and Automated Vehicles using Traffic Signal Information
Future roadways will rely on connected and automated vehicles (CAVs) to reduce traffic congestion, maximize fuel economy, and increase safety. Wang’s team plans to develop a novel method to produce the best speed profile using traffic signal phase and timing data. Successful completion of this project would result in a new method for real-time optimal control of CAVs. This work is essential to mainstream the use of CAVs for future transportation systems.
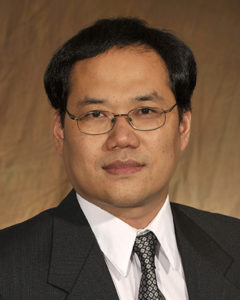
Xiaopeng Zhao
Department of Mechanical, Aerospace and Biomedical Engineering
A Multisensory Brain-Computer Interface for Intelligent Driving
Smart cars and intelligent driving have moved into the forefront of vehicle technology, opening up a number of areas of research. Zhao’s team proposes to investigate intelligent driving through the development of an interface designed to communicate information about a vehicle’s driving conditions to the driver.
2019 – 2020 Awardees
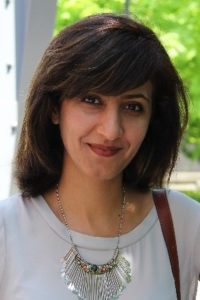
Mahshid Ahmadi
Department of Materials Science and Engineering
Machine Learning Driven Experimental Approach for the Discovery of New Organic-Inorganic Halide Perovskites for Optoelectronic Applications
Organic-inorganic halide perovskites have emerged as materials of choice for low-cost photovoltaics and optoelectronics due to relatively easy solution synthesis and unique spectrum of functional properties. The objective of this project is to establish a machine learning based experimental approach towards discovery and prediction of hybrid perovskites properties via combinatorial synthesis and evolutionary experiment optimization.
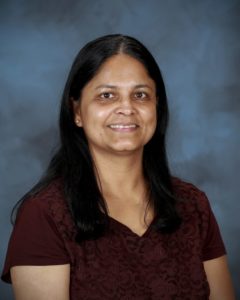
Sindhu Jagadamma
Department of Biosystems Engineering and Soil Science
Is Soil Manganese a Major Driver for Organic Carbon Cycling in Croplands?
Manganese (Mn), an essential plant micronutrient, is believed to play a critical yet poorly understood role in terrestrial ecosystem carbon (C) cycling, particularly in human manipulated agoecosystems. Her team proposes a series of field and laboratory experiments that simulate excess Mn mobilization in agricultural soils to quantitatively assess the role of Mn in ecosystem C cycling. The findings of this proposed study will inform whether acidity-induced elevated Mn availability is a critical driver in soil organic C storage/loss in highly managed agroecosystems.
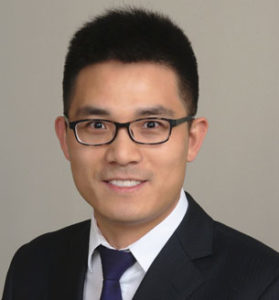
Zhenbo Wang
Department of Mechanical, Aerospace and Biomedical Engineering
Real-Time Control for Connected and Automated Vehicles using Traffic Signal Information
Future roadways will rely on connected and automated vehicles (CAVs) to reduce traffic congestion, maximize fuel economy, and increase safety. Wang’s team plans to develop a novel method to produce the best speed profile using traffic signal phase and timing data. Successful completion of this project would result in a new method for real-time optimal control of CAVs. This work is essential to mainstream the use of CAVs for future transportation systems.
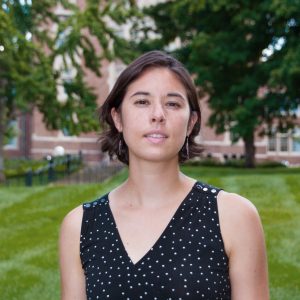
Constance Bailey
Biochemical and Computational Probing of Mutant-Biocatalyst Relationships in Polyketide Synthase Ketoreductases
Carbonyl reduction is a commonly used biocatalytic transformation in the manufacture of chiral pharmaceutical intermediates and other high value chemicals. Bailey’s lab has focused on developing ketoreductase (KR) domains from bacterial biosynthetic enzymes as a model for developing stereoselective biocatalysts. Her team plans to use computational and experimental methods to probe mutant-biocatalyst relationships in these enzymes; work that has applications in pharmaceutical and chemical manufacturing.
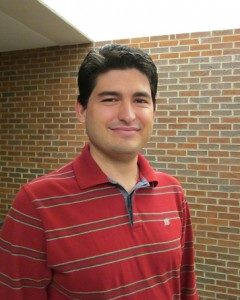
Francisco Barrera
Department of Biochemistry & Cellular and Molecular Biology
Stimuli-responsive Neuromorphic Computing
Neurons in the human brain achieve a breadth of computing capabilities that are superior to man-made computers. Neuromorphic computing seeks to create computing systems inspired by the firing of ion channels as the synapse that connects neurons. However, current neurotrophic computing systems are underwhelming in their efficiency, versatility, and energy consumption. Barrera’s team hopes to address this by developing improved membrane networks to encourage more versatile and responsive neurotrophic computing systems.
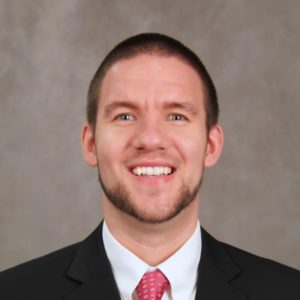
Hugh Medal
Department of Industrial and Systems Engineering
Machine-Learning-Enabled Modeling for High-Dimensional Dynamics of Materials Processing
Understanding the movement of ions through material is crucial to understanding important properties such as radiation, stress cracking, and ion conductivity. Because of this, there is an urgent need to map high-dimensional energy landscapes (HDEL). Medal’s team plans to address this need via machine-learning-enabled modeling.
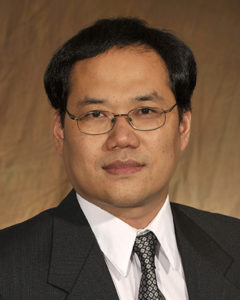
Xiaopeng Zhao
Department of Mechanical, Aerospace and Biomedical Engineering
A Multisensory Brain-Computer Interface for Intelligent Driving
Smart cars and intelligent driving have moved into the forefront of vehicle technology, opening up a number of areas of research. Zhao’s team proposes to investigate intelligent driving through the development of an interface designed to communicate information about a vehicle’s driving conditions to the driver.